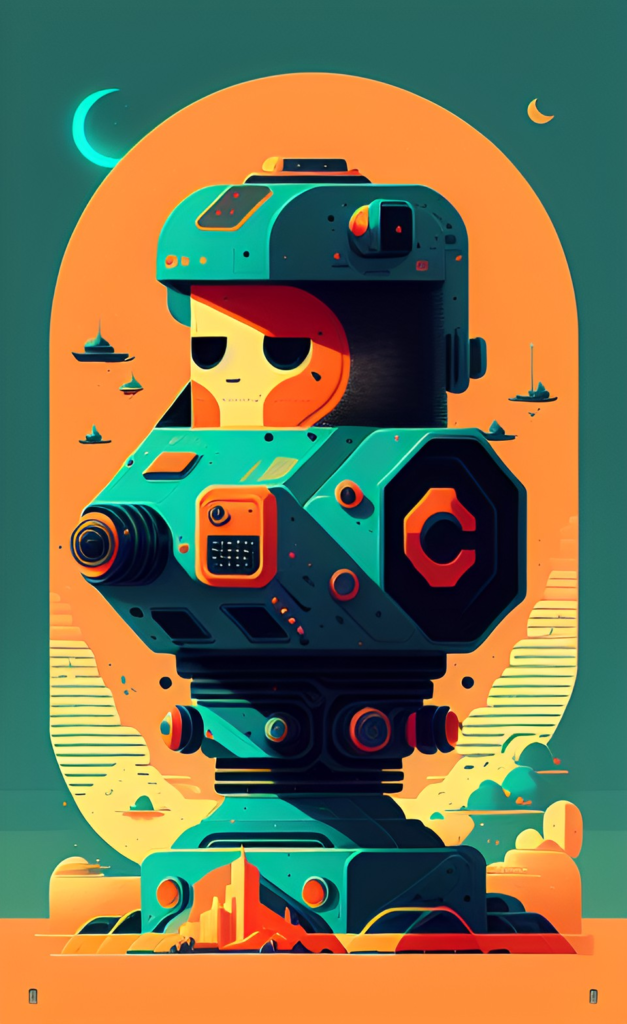
On one hand, the field of “machine learning” has gained significant attention in recent years, sparking debates about the nature of machines’ ability to truly “learn.” This essay challenges the notion that machine learning involves genuine learning and argues that it is primarily a statistical model adjustment process. It cautions against anthropomorphizing machines and falling into linguistic traps that create a misleading perception of intelligence. The essay also explores the limitations of terms like “hallucination” and proposes an alternative perspective on the discipline.
Body:
- The Illusion of Learning: Machine learning, at its core, involves adjusting weights within a statistical model to optimize performance. While this process can generate impressive results, it differs fundamentally from how humans learn. Teaching a child involves comprehension, reasoning, and the ability to generalize knowledge, which are not encapsulated in the adjustment of model weights. Therefore, referring to machine learning as “learning” can be misleading.
- The Pitfalls of Anthropomorphizing: The use of terms like “AI hallucination” and attributing human-like qualities to machines creates an illusion of intelligence. This anthropomorphization leads to misconceptions about the true capabilities of machines. By unconsciously ascribing characteristics such as understanding or knowing to AI systems, we inadvertently deceive ourselves and inflate their capacities beyond what they truly possess.
- The Slippery Slope of Language: Even the use of pronouns like “I” by chatbots can blur the line between human and machine intelligence. This linguistic choice can subtly reinforce the notion of a conscious entity behind the keyboard, fostering the illusion of a human-like conversation partner. However, it is crucial to remember that chatbots are ultimately algorithms designed to simulate human-like responses, rather than possessing genuine understanding or consciousness.
- A Shift in Perspective: Instead of using the term “AI” with its potential for misinterpretation, an alternative suggestion is to refer to this discipline as “applied statistics.” By emphasizing the practical application of statistical methods, we ground our understanding in a more accurate representation of the field’s capabilities. Recognizing the automation potential of applied statistics can help us focus on its ability to alleviate mundane tasks and streamline processes, as described by David Graeber in his book on “bullshit jobs.”
On the other hand while there is an overlap between machine learning and applied statistics, it is important to note that machine learning is a subfield of applied statistics rather than a complete synonym. Applied statistics encompasses a broader range of statistical methods and techniques applied to various fields, including traditional statistical analysis, experimental design, and modeling. Machine learning, on the other hand, focuses specifically on developing algorithms and models that allow computers to learn patterns and make predictions or decisions without being explicitly programmed.
Machine learning techniques often involve statistical methodologies, such as regression analysis, clustering, or classification algorithms. However, machine learning goes beyond traditional statistical inference by utilizing computational power to process vast amounts of data and automatically adjust model parameters based on observed patterns. This adaptability and ability to learn from data are distinguishing characteristics of machine learning.
So, while applied statistics and machine learning share common ground, the latter extends beyond traditional statistical approaches by incorporating advanced algorithms and automated learning capabilities. Machine learning is a specialized field within the broader domain of applied statistics, aimed at developing intelligent systems capable of learning and making predictions from data.
automated learning is still applied statitics
While automated learning, as seen in machine learning, has its roots in applied statistics, it is important to recognize that machine learning expands upon traditional statistical methodologies to incorporate computational techniques and algorithms specifically designed for data-driven learning and pattern recognition.
Applied statistics traditionally focuses on analyzing and interpreting data using established statistical models and techniques. It involves hypothesis testing, regression analysis, experimental design, and other statistical methods to draw conclusions and make inferences about a population based on sample data.
Machine learning, on the other hand, aims to develop algorithms that enable computers to automatically learn patterns and make predictions or decisions without being explicitly programmed. It involves the creation of models that can learn from data, identify complex relationships, and make accurate predictions or classifications.
Machine learning algorithms, such as neural networks, decision trees, and support vector machines, use statistical concepts as a foundation. However, they often go beyond traditional statistical methods by incorporating optimization algorithms, computational techniques, and advanced mathematical concepts.
While applied statistics and machine learning are intertwined, machine learning represents an expansion and specialization of statistical techniques to enable automated learning from data. It leverages computational power, algorithmic complexity, and large datasets to develop models capable of learning and making predictions in complex and high-dimensional spaces.
In summary, while machine learning has its roots in applied statistics, it encompasses a distinct set of techniques and methodologies that go beyond traditional statistical analysis, focusing on automated learning and predictive modeling from data.
Conclusion: The illusion of “machine learning” as true learning and the temptation to anthropomorphize machines are challenges we face in understanding the capabilities of artificial intelligence. By critically examining the language we use and avoiding linguistic traps, we can develop a more realistic perspective on the field. Reframing the discipline as “applied statistics” highlights its practicality and automation potential, allowing us to appreciate its ability to tackle mundane tasks and enhance efficiency.